Advancements in Mine Action: Enhancing Remote Reporting and Analysis Through Innovative Technologies
CISR JournalThis article is brought to you by the Center for International Stabilization and Recovery (CISR) from issue 28.3 of The Journal of Conventional Weapons Destruction available on the JMU Scholarly Commons and Issuu.com.
By Rory Collins [ United Nations Office for Project Services ], Lionel Fragniere [ Swiss Armed Forces International Command ], and Mateo Dulce Rubio [ Carnegie Mellon University ]
This paper examines the innovative initiatives undertaken by the United Nations Office for Project Services (UNOPS) Peace and Security Cluster (PSC) in advancing humanitarian mine action (HMA) through remote reporting and analysis technologies. It provides an in-depth analysis of key initiatives, including the development of a mapping tool in Gaza, the application of Natural Language Processing (NLP) for efficient data management in improvised explosive devices (IED) threat mitigation globally, and the utilization of advanced remote sensing and machine learning technologies in Syria and Afghanistan. Through these use cases, we demonstrate how innovative technologies can be incorporated into HMA to increase efficiency and safety, while also outlining future plans and the challenges faced in these initiatives.
Introduction
The UNOPS PSC has the largest operational mine action capacity within the UN system, implementing on behalf of partners including the United Nations Mine Action Service (UNMAS), the United Nations Development Programme, and the United Nations Office of Counter-Terrorism. To optimize operational efficiency and effectiveness, UNOPS strives to be at the forefront of integrating innovative technologies into mine action. Furthermore, as a senior service provider for UNMAS, UNOPS PSC designed, implemented, and manages the Global Information Management System (IMS) used to monitor and report on operations. This paper provides an analysis of UNOPS’ key initiatives in remote reporting and analysis, detailing their practical applications, the challenges encountered, and future developmental plans. The goal is to provide a resource for the wider mine action community, illustrating how these technologies can be leveraged to improve mine action efforts globally.
Concretely, this paper outlines three key areas of innovation advanced by the UNOPS PSC to increase efficiency and safety in HMA efforts. First, automated data-sharing protocols that integrate diverse data sources such as satellite imagery, survey data, and historical records provide comprehensive, real-time information for operational planning in Gaza. Second, the application of Natural Language Processing (NLP) allows us to leverage and integrate the available reports on IEDs into the UNMAS Global IMS for IED threat mitigation. Finally, the paper discusses the use of remote sensing technologies to create 3D models of rubble in Syria, as well as the assessment of socioeconomic impacts using satellite imagery and artificial intelligence (AI) in Afghanistan.
The paper concludes by discussing the way forward to establish stronger collaborations with leading universities and technology partners to integrate state-of-the-art data science methodologies into mine action initiatives.
Automated Data-Sharing Protocols for Comprehensive Operational Planning
Context. The scale of the explosive ordnance (EO) problem in Gaza is anticipated to be so great that it is already significantly impacting how humanitarian actors can access areas.
To mitigate any risks posed by EO, explosive ordnance disposal (EOD) officers have been requested to join all humanitarian aid missions in the middle and northern parts of Gaza. These mission planning processes are based on many prioritization factors, including access, threat assessments, and impact.
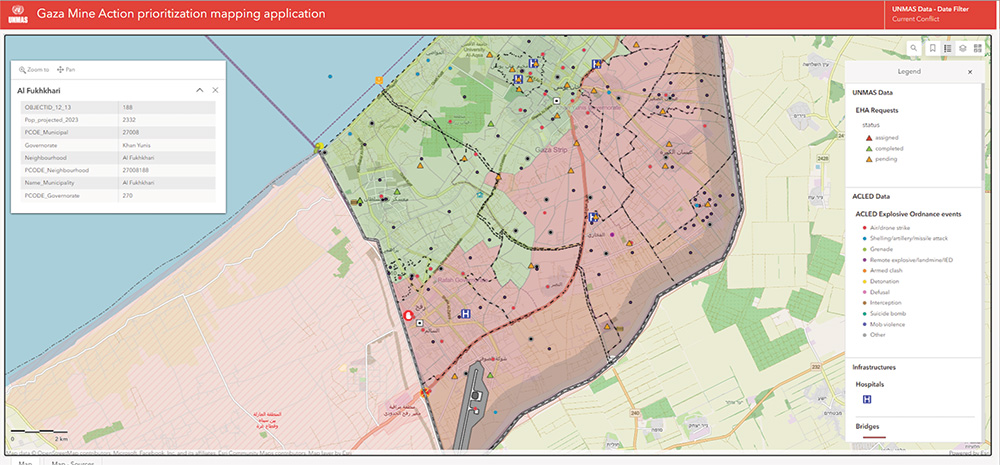
To assist in these processes, the UNOPS has developed an innovative planning and prioritization mapping tool that provides EOD officers with real-time access to relevant decision-making data. This tool integrates multiple data sources from various partners, including satellite imagery, survey data, and historical records to provide comprehensive, real-time information for operational planning.
Approach. The approach involves coordination with partners to acquire relevant data, discuss data-sharing protocols to inform EOD activity planning, conduct quality checks, and map multiple reporting schemas. Data is collected from various media, including Esri Distributed Collaboration and custom-made extract, transform, and load tools using Python scripting and application programming interfaces to facilitate EOD activity planning through multiple platforms. Regular quality checks ensure the validity and accessibility of the information while continually watching for relevant data from partners and open sources.
Results. The UNOPS mapping tool has significantly improved the operational planning capabilities of EOD officers in Gaza. By automating data sharing and integration, it streamlines the gathering and processing of critical information, allowing EOD officers to focus on strategic planning.
This tool provides real-time situational awareness by integrating satellite imagery, survey data, and historical records into one platform. EOD officers can quickly assess threats, prioritize areas, and plan missions with greater accuracy. Access to updated information is crucial in volatile environments.
Significantly, the tool also improves coordination among humanitarian actors and mine action partners. Serving as a central hub for EOD officers, it ensures all stakeholders have access to high-quality information, facilitating cohesive efforts. Its interoperability with systems such as the United Nations Office for the Coordination of Humanitarian Affairs’ (OCHA) DataHub improves partnership efficiency and effectiveness.
Challenges. The integration of diverse data sources requires robust data management frameworks to ensure compatibility. Sustaining real-time data flow demands significant technical resources and infrastructure to support continuous data streaming and processing. Ensuring the quality of information is critical, as all datasets must be harmonized to integrate correctly with other sets presented.
Future Plans. UNOPS views mapping tools like these as hubs within a network of mine action partners, and not just siloed platforms. The automated interoperability of these tools with other systems, such as OCHA’s DataHub, is changing the landscape of partnerships in mine action.
Looking ahead, predictive analytics will further enhance the tool. By leveraging historical data trends and real-time inputs, it can improve forecasting of potential EO contamination, enabling proactive measures and improving long-term planning and risk mitigation.
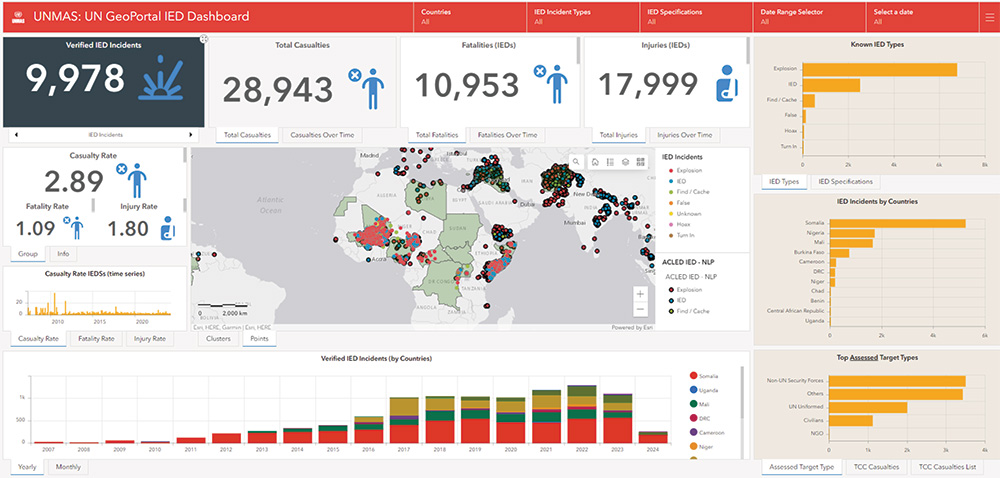
Application of Natural Language Processing for Improvised Explosive Device Threat Mitigation
Context. Developed and managed by UNOPS PSC for its partner UNMAS, the Global IMS is an Esri enterprise-based GIS-centric platform for the reporting and analysis of hundreds of mine action activities for over seventeen mine action country programs.
IED reporting is one of the workflows on the IMS needed for well-informed IED threat mitigation efforts, where access to information and its accurate processing is critical. To leverage the full extent of available IED reports, UNOPS implemented advanced NLP techniques to streamline the processing of large volumes of data from multiple sources, thus enhancing the accuracy and efficiency of threat assessments. NLP scripts are utilized to automatically detect, clean, and catalog reports from a variety of sources, resulting in highly accurate and comprehensive datasets.
Approach. Data is obtained from various sources using custom methods. Relevant data is extracted from the datasets with predefined definitions via structured query language queries and NLP-enabled Python scripts.
The datasets are then harmonized, cleaned, and sometimes combined to accommodate their specific functions. NLP scripts are applied to the datasets, with models trained from existing in-house datasets containing validated information and regularly enriched with new data. Although the models return a high level of accuracy, human involvement is key to ensuring the overall validity of the data.
Results. The application of NLP in the UNMAS IMS has significantly enhanced IED threat mitigation efforts. By automating the detection, cleaning, and cataloging of IED reports from various sources, the system has improved both the accuracy and efficiency of threat assessments.
The integration of NLP scripts has streamlined data processing, allowing for the rapid and accurate extraction of relevant information from large datasets. This automation has reduced the time and resources previously required for manual data handling, enabling faster and more informed decision making. The result is a comprehensive and highly accurate dataset that supports effective IED threat mitigation.
NLP techniques have also facilitated the harmonization of data from diverse sources, including governmental and nongovernmental organizations (NGOs), each with different formats and languages. This capability ensures that data is consistently processed and integrated into the IMS, providing a unified platform for reporting and analysis. The models, trained on validated in-house datasets, achieve high accuracy, though human oversight remains essential to maintain data validity.
Challenges. Managing data from various sources, including governmental and NGOs, each with distinct formats and languages, is a primary challenge. Additionally, NLP must effectively process data across multiple languages and dialects, which vary significantly in syntax and semantics.
Future Plans. Future efforts will focus on enhancing NLP algorithms to better handle complex linguistic variations and diverse data formats, thereby improving processing speed and data accuracy. The integration of automated translation capabilities is planned to enable the efficient processing of multilingual data, broadening the system’s applicability in international contexts.
Use of Remote-Sensing Technologies in Syria and Afghanistan
Context. The HMA sector faces increasing challenges in identifying and clearing landmines and unexploded ordnance (UXO) in conflict-affected areas worldwide. Adopting remote-sensing technologies, such as AI and satellite imagery, is crucial as these tools can enhance the accuracy and speed of detecting hazardous areas. This technological integration not only ensures the safety of demining personnel by reducing their direct exposure to dangerous zones but also accelerates the clearing process, allowing for quicker restoration of safe environments for local communities.
As conflicts and the resulting contamination continue to evolve, the integration of advanced technologies becomes imperative to address these complex scenarios.
Approach. UNOPS has developed two advanced remote sensing and AI technologies for diverse applications: creating 3D models of rubble in Syria to help inform planning and prioritization, and evaluating socioeconomic impacts in Afghanistan aligned with the UN Sustainable Development Goals (SDGs).
In Syria, 3D modeling of the environment based on stereo imagery returns a point cloud similar to what light detection and ranging (Lidar) would do. The process is enhanced by using off-nadir angles and shadows from the imagery to obtain accurate building heights and deduce the volume of rubble, aiding in accurate debris assessment and safe removal operations. The ability to run these assessments remotely and automatically has a significant impact on the safety of EOD officers, as well as the time and resources needed to more accurately inform the critical and extremely dangerous non-technical survey operations in the field. This 3D visualization can also be used to plan operations with improved situational awareness and run change detection models for building, agricultural, and road damage detection.
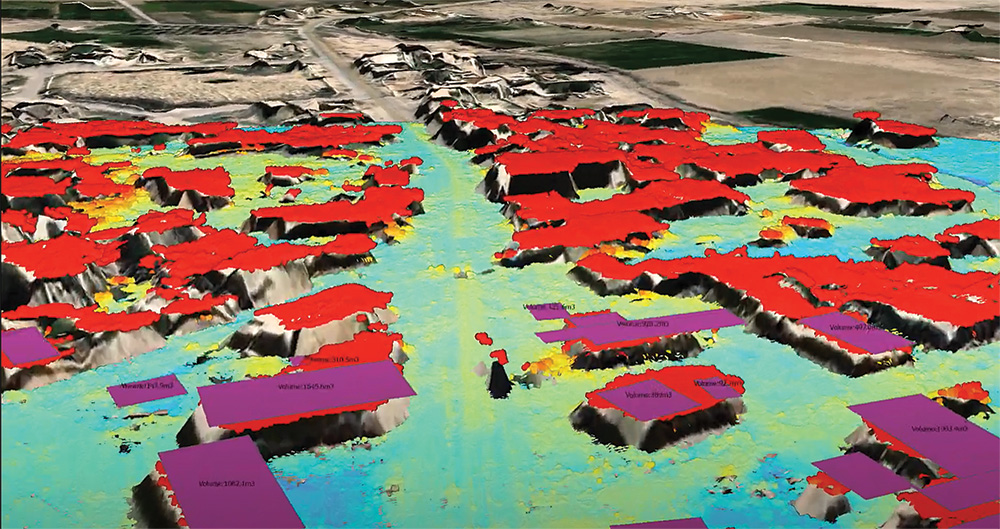
Afghanistan. AI models developed by UNOPS in collaboration with the Massachusetts Institute of Technology and regional partners such as Alcis, as well as high resolution satellite imagery from Maxar provided through strong partnerships with the US Department of State, are used to detect and measure positive socioeconomic changes resulting from HMA activities. This approach provides evidence of how UNOPS has enabled the development of a US$1 billion economic zone, including direct and indirect employment of over 15,000 people. This also provides the mine action program in Afghanistan measurable indicators that directly contribute to the UN SDGs, as well as evidence to support advocacy and further funding initiatives.
Challenges. Maintaining high data accuracy is essential for reliable threat assessments and planning, necessitating continuous validation and calibration of remote sensing tools. Developing AI models that are resilient to diverse environmental conditions and data quality variations remains a critical challenge.
Future Plans. Efforts will focus on advancing remote sensing technologies to include real-time monitoring, providing continuous updates on the status of mine-affected areas. Future plans include the development of AI-driven predictive models to forecast contamination and socioeconomic impacts, facilitating proactive planning and resource allocation. For example, these models could help forecast contamination risks by analyzing historical data, conflict intensity, and terrain features, allowing for targeted demining and resource allocation. Additionally, they could help assess the socioeconomic impacts of demining and infrastructure investments, predicting how these activities will affect local economies and communities. This proactive approach could contribute to safer operations, more efficient use of resources, and strategic investment in areas poised for significant growth.
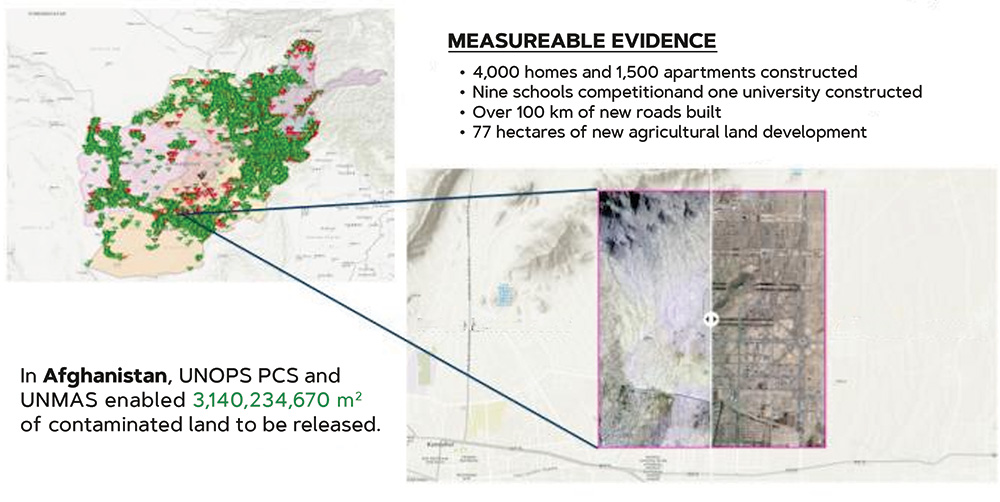
The Way Forward: Stronger Collaborations with Leading Universities and Technology Partners
UNOPS PSC Information Management and Analytics Team has established a collaborative innovations group dedicated to advancing technological solutions in mine action through strategic partnerships. This group collaborates with leading universities and technology partners to explore and implement cutting-edge innovations. Benefits of this relationship can be seen almost immediately, creating a direct channel from the innovative technology developed by academia to the mine action operations personnel making critical decisions in the field.
A recent example is the collaboration with researchers from Carnegie Mellon University to build an AI-informed system that estimates the probability of EO contamination in a given hazard polygon. The developed tool can be integrated into existing planning workflows to support the identification of priority areas for mine action land release activities in Afghanistan and globally.
Approach. Historical information on finalized land release operations is used to predict whether a new hazard area will be confirmed to be contaminated or canceled. A comprehensive set of relevant variables, including geographic attributes, socio-demographic characteristics, and remnants of conflict indicators is collected.
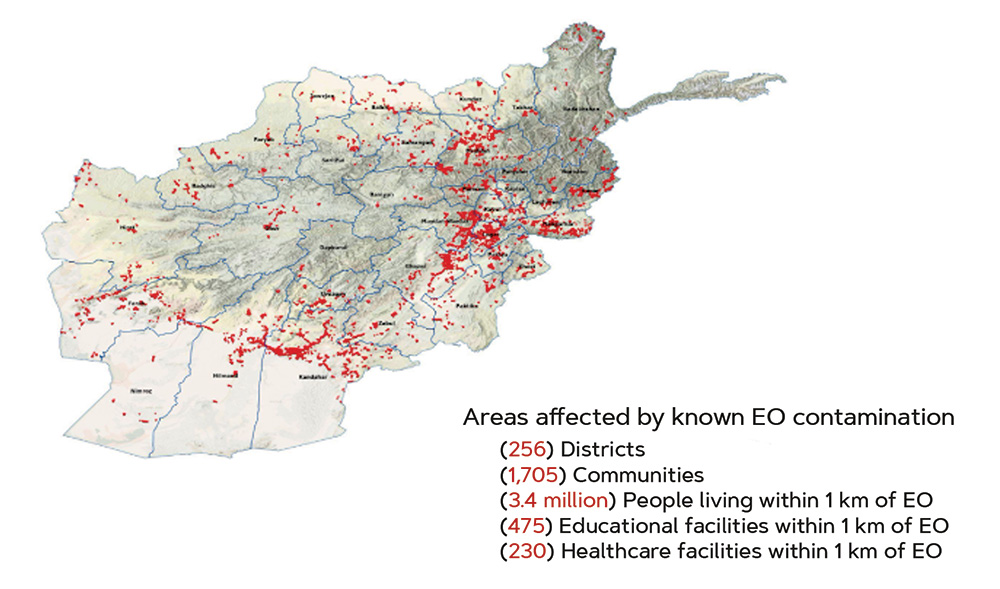
An AI model trained on this data learns the relationships between the geographic predictive features and historical EO contamination. The model is then extrapolated to new hazard areas to generate risk maps and identify the areas more likely to be contaminated.
The AI pipeline is tailored to the operations teams’ needs, estimating the risk of EO contamination in active hazard areas by collecting relevant variables adapted to the Afghan context, training the models with local historical data, and presenting the results in a user-friendly manner.
Results. The final model is a weighted average of Random Forest,1 LightGBM,2 and a neural network specifically designed for EO contamination identification3 (see Figure 6). The AI model maximizes the tradeoff between identified confirmed hazards and canceled false alarms. Among the top 10 percent of the polygons in the validation data with the highest estimated risk scores, 99.07 percent were correctly identified as contaminated hazards.
Each active hazard in Afghanistan is then assigned with an estimated probability of EO contamination that categorizes the polygons into areas of low, medium, high, and very high risk of EO contamination. The predicted risk score can then be used to support decision making and prioritization.
The model also outputs the most relevant features used to estimate the risk score for each polygon using SHAP values.4 SHAP values, or SHapley Additive exPlanations, are a tool used in the field of machine learning to explain the output of any machine learning model. They help clarify which features in a dataset are most important in a model’s predictions, providing transparency about how individual factors contribute. This is critical to ensure the interpretability and credibility of the system.
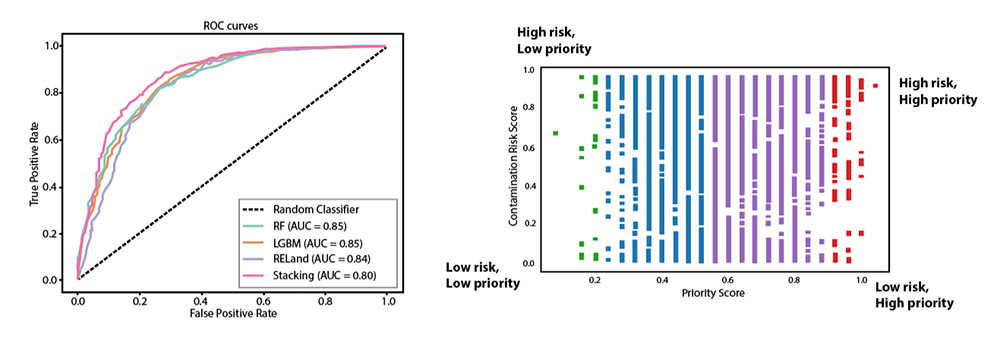
Challenges. Keeping abreast of rapid technological advancements and integrating new technologies into existing frameworks is a continuous challenge. Ensuring sufficient resources and funding to support ongoing innovation and development initiatives is critical for sustained progress.
Future Plans. Plans include expanding partnerships with academic and industry leaders to leverage a broader range of expertise and technological innovations. Increased investment in emerging technologies such as machine learning is anticipated to enhance data security, operational transparency, and overall efficiency in mine action activities.
Conclusion
UNOPS’ innovative approaches in remote reporting and analysis are transforming its mine action operations, providing enhanced efficiency and safety. Through continuous R&D and strategic collaborations, UNOPS looks forward to advancing the field further, offering practical, impactful solutions that benefit the global mine action community.
See endnotes below.
Rory Collins is an Information Management and Analytics Advisor at United Nations Office for Project Services (UNOPS), specializing in innovation and technology integration in humanitarian mine action. With a focus on enterprise system implementation and management, as well as remote sensing, Collins has led multiple projects with the United Nations that enhance operational efficiency and innovation in humanitarian mine action (HMA).
Lionel Fragniere is a Senior Technical Officer with the Swiss Armed Forces International Command, supporting UNOPS with extensive experience in information management, geographic information system, and artificial intelligence applications within HMA. His work primarily focuses on developing advanced tools for operational planning and improving the accuracy of threat and damage assessments through innovative technologies.
Mateo Dulce Rubio is a PhD candidate in Statistics and Public Policy at Carnegie Mellon University. His research focuses on developing flexible and robust methods inspired by challenges arising in post-conflict scenarios and peacebuilding efforts, using tools from nonparametric statistics, casual inference, and responsible machine learning.